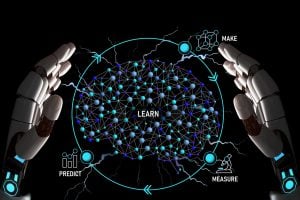
Bringing together material and data scientists, the Johns Hopkins Applied Physics Laboratory (APL) in Laurel, Maryland, is leveraging the power of artificial intelligence to rapidly discover materials that can withstand extreme environments.
“As the U.S. faces pressing national security challenges, there are increasing operations in austere environments — and those operations require revolutionary new materials,” said Morgan Trexler, who leads APL’s Science of Extreme and Multifunctional Materials program. “We cannot wait decades to discover materials that meet those needs. By infusing AI approaches throughout the discovery process, we can more quickly and intentionally identify materials for complex, specific applications.”
Because researching new materials is time-consuming and expensive, Trexler added, researchers tend to identify and augment shortcomings in existing materials, rather than explore new element combinations from scratch.
“The approach to building on existing materials will only ever yield limited improvements,” said Keith Caruso, chief scientist in APL’s Research and Exploratory Development Department. “To create groundbreaking materials, we need to make a fundamental leap.”
Turning Up the Heat
Caruso leads an APL project that is harnessing improvements in AI and machine learning to find materials that can withstand extreme heat as components of a hypersonic vehicle.
“There are a huge number of structure and element combinations for potential materials,” said Victor Leon, an AI research scientist on the project. “It would be impossible to effectively explore that massive space using a purely experimental and computational approach. But, by combining AI, high-fidelity density functional theory simulations and material science knowledge, we can narrow the search to a more palatable set of combinations.”
The team’s focus on applications for high-temperature capabilities drove the creation of a novel AI architecture for materials discovery that can target desired properties, structures and chemistries. Once the search is refined, APL researchers create prototypes and use high-throughput screening to rapidly measure material characteristics. The process is repeated over and over, each time feeding new information into the AI model to provide more predictive results.
“Materials discovery is really a three-part problem,” said Christopher Stiles, chief scientist of APL’s Electrical and Mechanical Engineering Group. “First, you need to discover the potential composition and structure. Then you need to discover how to synthesize the material. Finally, you need to measure relevant properties and, if properties meet desired values, understand what compositional, structural or processing aspect, or combination thereof, is responsible for that unique behavior you didn’t have yesterday.”
The project is one of several at APL targeting the discovery and development of materials with unprecedented properties that can withstand extreme environments.
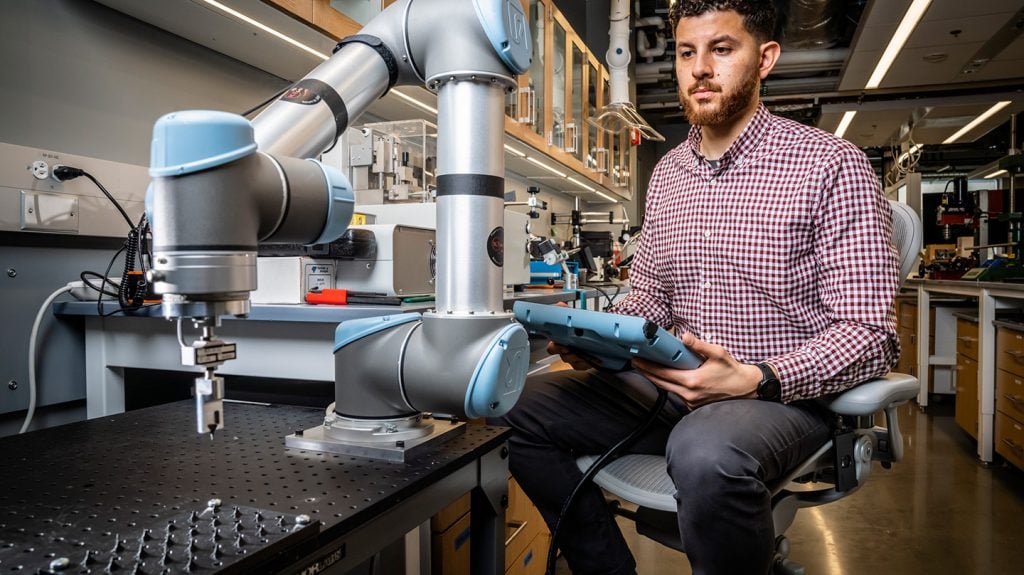
Sal Nimer leads the development of a system that uses a robotic arm to test properties under extreme conditions.
Credit: Johns Hopkins APL
Unprecedented Material Properties
An APL team is developing a framework, named Autonomous Researcher for Materials Discovery (ARMD), to directly embed mission requirements as part of the materials discovery process. Currently, the team is working on accelerating the development structural refractories for high-temperature applications.
“Testing properties of new materials is typically a slow process that creates a bottleneck between discovery and application, particularly for extreme environments like elevated temperature,” said Sal Nimer, a senior materials and test engineer and the principal investigator on ARMD.
Nimer’s team is creating samples using an additive manufacturing process known as blown powder directed energy deposition. This approach enables fabrication of hundreds of unique samples, with simultaneous variations in composition and processing conditions, on a single additive manufacturing build plate in custom-designed sample geometries tailored for mechanical testing.
The build plate containing hundreds of unique samples is then autonomously tested using an instrumented robotic arm. To understand properties under extreme conditions, a laser is attached at the end of the arm to heat the samples during testing. Depending on the details of the fabrication and test geometry, this specimen and method design enables up to three times less material volume, three times faster fabrication and five times faster testing than standard approaches.
Test data is then analyzed through a Bayesian optimization model, which suggests promising candidates and optimized parameters to meet the needs of each specific application. Overall, the ability to sample large, complex parameter spaces simultaneously and autonomously enables at least a 20-times throughput increase, significantly accelerating discovery and understanding.
In a separate project, materials scientists Eddie Gienger and Lisa Pogue created dozens of complex microstructures for multi-principal element alloys, which are stronger and better suited for materials operating in extreme environments. Gienger and Pogue developed a pathway that links alloy phases — distinct materials formed when an alloy is heated or cooled — to their mechanical properties. The capability provides a way to quickly create and analyze thousands of local data within bulk samples to intelligently inform the design of new materials and enable rapid material discovery.
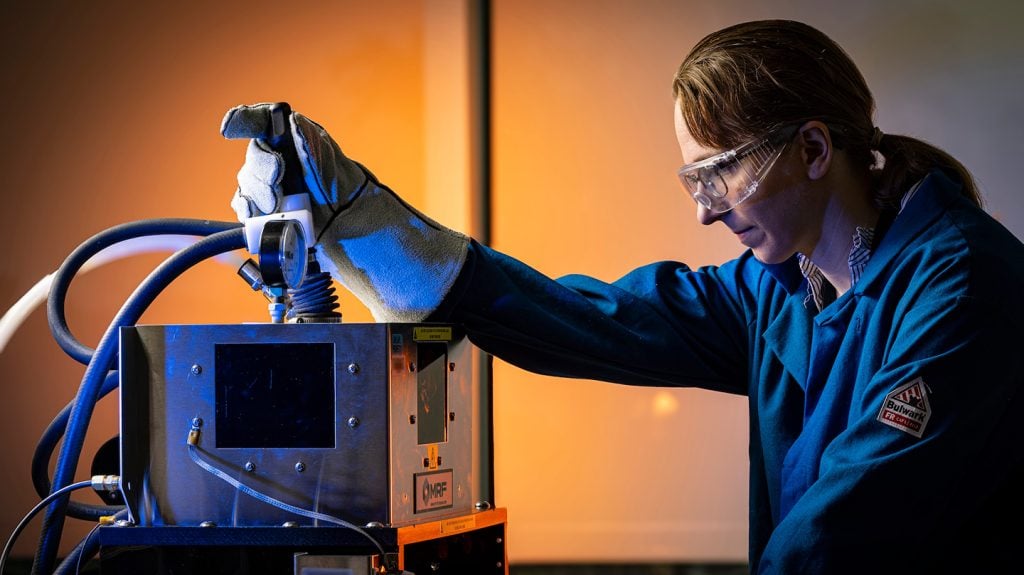
Lisa Pogue uses an arc melter to melt an alloy of zirconium and titanium. The process is part of the framework to link alloy phases — distinct materials formed when an alloy is heated or cooled — to their mechanical properties.
Credit: Johns Hopkins APL/Ed Whitman
Researchers across APL have accelerated other testing methods too, across a range of loading modes and properties, including X-ray diffraction and X-ray computed tomography, to quickly assess tensile strength, porosity-induced stress fields, fatigue resistance and corrosion performance. These methods provide valuable material information that is otherwise time-consuming and arduous to obtain. By advancing materials characterization, data can be brought into the discovery process earlier, more rapidly and in larger quantities, to accelerate the path to materials discovery, demonstration and final implementation.
Finding Alternatives
Another national security challenge is the supply chain and limited availability of critical materials. Rare earth elements in particular have a high processing cost and have been identified as a high supply risk by the Department of Energy. Currently, magnetic rare earth elements are vital to future green energy and electric technologies, including electric vehicles, so alternative solutions are needed.
“As electric vehicle use soars, there is a significant need for lower-cost, more abundant magnetic materials that don’t require rare earth elements,” said Nam Le, a computational physicist at APL. “It’s easy to tell if a new candidate material is free from rare earth elements, but it’s difficult to know if it would make a good magnet, so that’s the part we’re focusing on screening.”
Le leads De-Risking Key Materials in Energy Technologies (DRK MATER), a project to develop models to discover materials that can satisfy multiple requirements, such as strong magnetic properties and lower logistical and environmental impact.
For permanent magnets, there are two important properties: saturation magnetization and coercivity, which is a measure of the resistance of material to becoming demagnetized. However, AI models that can predict saturation magnetization don’t work as well to predict coercivity.
“When you start asking an AI model about a material it wasn’t trained on, the results can be very wrong,” said Le. “Initially, we got great results for one property but terrible, inaccurate results for the other.”
To improve the models, Le added, APL is working on methods that can learn from different sources simultaneously: augmenting small numbers of experimental data points with large numbers of data points simulated using a physics-based method called density functional theory, along with constraints related to supply risk.
Making Materials for the Moon, on the Moon
The need to utilize readily available resources for material creation extends beyond Earth. As the U.S. progresses with its Moon to Mars strategy, AI-guided discovery for in situ materials could one day enable lunar and planetary missions.
Stiles leads an APL team collaborating with Tyrel McQueen at the Johns Hopkins Krieger School of Arts and Sciences and Mark Foster at the Johns Hopkins Whiting School of Engineering to develop machine-learning tools that accelerate targeted materials discovery and manufacturing. The project could help researchers discover, design and fabricate new materials in austere environments such as the Moon.
Building on APL’s AI-enabled predict-make-measure approach, which was successfully employed in the targeted discovery of a novel superconductor, the team extended the approach to utilize large language models. These models were used to create a comprehensive knowledge base of elements found on the lunar surface. Because there are no comprehensive databases of lunar constituents or lunar manufacturing processes, the team is also developing these foundational capabilities.
The team leveraged generative AI to create millions of candidate materials and physics-based AI to down-select materials for their synthesizability. Then, neural networks are used to predict mechanical properties of interest, from which a subset is further down-selected. From that reduced set, the team created over 200 samples using directed energy deposition and characterized them to improve and retrain the AI models.
“While we account for restrictions to building structures on the Moon, there are factors that could benefit manufacturing on the lunar surface. The Moon’s microgravity environment surrounded by a vacuum could make processes that are difficult on Earth easier to accomplish,” said Milena Graziano, a senior materials scientist at APL. “There are a lot of different levers to pull that could be exploited for operating in an extreme environment.”