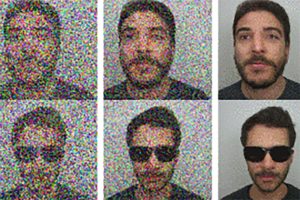
Facial recognition software works best with images captured in daylight but has difficulty with visuals taken in darkness or low light using thermal or infrared cameras. Converting these images into clear photos for accurate facial verification is challenging and requires a process called Thermal-to-Visible (T2V) image translation.
Johns Hopkins engineers have devised a way to improve facial recognition in these low-light situations. Their Denoising Diffusion Probabilistic Model (DDPM) can convert thermal images into clear, photo-like ones, helping to ensure that facial verification is accurate even in situations where there isn’t much light available. The team’s results appeared in IEEE Explore.
“Modern surveillance systems rely on the ability to use facial recognition to identify individuals, but it becomes challenging to recognize faces in low-light or night-time conditions when images must be taken using thermal cameras,” says Vishal Patel, an associate professor of electrical and computer engineering and faculty member at Johns Hopkins Engineering for Professionals program. “Our system works to translate those thermal images into visible ones that can be effectively used.”
Patel and Nithin Gopalakrishnan Nair, a PhD student in electrical and computer engineering, used a diffusion process to train their model. They showed the system pairs of images—one, a normal photo, and the other, a thermal image of the same scene. By comparing the pairs, the system learned to understand the “relationships” between regular photos and thermal images, learning how features such as faces look different across the two image types. The result is a system that understands how to turn a thermal image into a visible one that can be interpreted by facial recognition systems.
“The DDPM sampling process starts with a noisy image, conditioned with the image produced by thermal cameras, and then uses a series of steps to clean it up or ‘denoise’ it. By the time it has finished, you have a clear, visible image ready for facial verification,” Nair said.
Because this method is stable and more accurate than existing approaches, it could make security systems more robust and dependable, the team says.
Interested in learning more about JHU Engineering for Professionals programs? Reach out to our admissions team today!