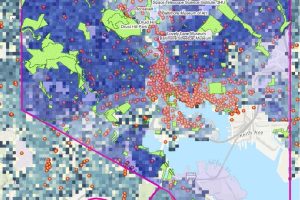
Using artificial intelligence and machine learning techniques, students in the Whiting School of Engineering’s Engineering for Professionals’ Information Systems Engineering program used their capstone project to study how environmental factors such as the number and location of fast-food restaurants, parks, and walkable spaces affect community residents’ health.
Team members included Khang Luong, DevSecOps engineer at Ricardo Defense in Troy, Michigan; Santosh Sharmasubedi, an IT specialist at the U.S. Department of Justice’s Office of the Inspector General in Washington, D.C.; and Alexander Wang, senior global supply manager for Woven by Toyota in Palo Alto, California. Their adviser was Lawrence Husick, instructor of the team’s Entrepreneurship, Innovation, and Corporate Success course.
What problem were you trying to solve?
“We wanted to address health epidemics and help consumers have an awareness of the hidden neighborhood environmental forces impacting their lifestyles and habits based on where they live. We believe revealing these forces at play in the consumers’ life can enable consumers to make smarter and more conscious decisions,” said Wang.
Additionally, the team sought to highlight the importance of quality datasets when using these types of modeling tools.
“As the saying goes, ‘Your predictions are only as good as your data.’ When quantifying the correlative effect of fast-food restaurants and park walkability within a geographical region, we needed to ask ourselves what was valid and what was just background noise. Developing this train of thought aids in future modeling that will follow the same path,” said Luong.
How did you use Information Systems Engineering to tackle this task?
Information systems engineering focuses on assisting humans in making high-level decisions based on synthesizing low-level data, said Wang.
“Our project uses machine learning techniques and CDC neighborhood data on obesity (based on Baltimore’s census tracts) and points-of-interest location data—fast-food restaurants, grocery stores, walkable streets, parks, and so forth—to train our model to predict health outcomes: in our case, one’s propensity to developing obesity. Using AI and ML components, our tool empowers the user to gain awareness of how proximity forces are shaping their probability for certain health outcomes,” he said.
The team used data instrumentation outside the context of computation to reveal findings that can affect real people.
“We demonstrated the function and value of a working baseline model of our proposed web tool that is low-cost and can scale. Such a tool that highlights the impact of proximity forces can benefit general users and policymakers by empowering users to have more information about important decisions such as potential health outcomes, particularly when making real estate decisions,” Wang said.
Luong adds that information systems engineering also focuses on building on the work of others to advance science and take technology “to new heights.”
“We used a very powerful suite of tools called ArcGIS which enabled us to not only overlay datasets like census tracts and restaurant coordinates but also the polygonal shape of parks. The engineering aspect however revolved around isolating the relevant data so that it can then be fed into the machine learning model. A combination of filters and calculations had to be performed and at one point when we had over 50,000 datasets that we then had to filter. As I said earlier, determining what is background noise and what is useful is a challenge, and that involves a systemic approach,” Luong explained.
What did you find out?
The research revealed that people who lived closer to fast-food restaurants and lived in communities where the walkability index was lower had higher body mass index (BMI) scores than did people who lived in neighborhoods with fewer fast-food restaurants and more walkable spaces, Wang said.
The team also learned what remarkable tools machine learning and artificial intelligence can be.
“I learned the vast application of AI and ML including the ways it can enable consumers to make better decisions and highlight the hidden forces at play in their lives. I discovered new ways to make AI/ML more accessible for the average user. Throughout the process, it was shown that policymakers will also be able to use these techniques to show statistical reasoning and graphically make a case to support location-based policy development projects that will result in increased public goods for society,” Wang said.
Luong said he also learned that the tools to model our lives are only as good as the engineers behind them and the countless statisticians who collect and process the datasets.
“ML and AI is the next frontier of technology and it has an unending hunger for quality data. Finding this data is no easy task and you need to be creative, determined, and unrelenting. Once you get the data you need to think, ‘How can value and meaning be abstracted out?’ Once those determinations are figured out then you see which model works best and what type of ML is appropriate to use,” he said.
What’s next? Will you continue working on this project?
Wang said that he proposed this concept after being inspired by a data analytics course demonstrating how proximity metrics can reveal hidden correlations, like reduced crime rates near libraries. His proof-of-concept tool highlights these hidden forces, but he wants to develop a more robust version for public and private stakeholders.
“This proximity model could be extended to predict opioid addiction risk based on the location of pain clinics or improve crime monitoring by identifying correlations between certain crime trends and a neighborhood’s proximity to certain establishments such as those selling alcohol. More applications include gaining support for public school investment by showing links between corporate HQ proximity to executives’ homes and school quality. Studies reveal higher profits when CEO homes are closer to corporate HQs. Studies also reveal CEO’s homes are correlated with higher quality schools, presumably due to spouse preferences, allowing real estate strategies to highlight public education funding’s correlation with rising home values,” he said.