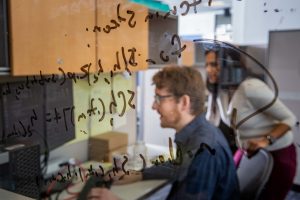
Inquisitive and determined are the attributes marked by students journeying to earn a data science or computer science master’s. These traits come in hand as, despite their vast knowledge in the sciences, working professionals are often challenged to keep up with the constantly changing nature of their fields.
To illustrate the branching out of Computer Science into Data Science, consider the evolution of the bicycle into the Peloton bike. The strong mechanics of the basic bicycle created the foundation for its transformation into the a-tech fitness phenomenon. Similarly, computer science is the essential foundation of data science, and as a collective unit, these sciences have furthered technology to incredible heights.
Understanding the differences between a master’s in data science versus computer science can be key when considering your next career move, as each program offers a different path and expertise.
Master’s in Computer Science Overview
The study of computer science at the master level is all-encompassing. The program provides advanced training in computational design, security, programming theory, architecture, applications, software development, and hardware. This comprehensive approach to computing offers insight into networks, operations, design parameters, and databases—with specialized topics. A computer science master’s degree provides graduates with a wide range of career paths in the information technology arena.
Get more detailed information about the degree, and review computer science specifics.
Master’s in Data Science Overview
Data science offers students the opportunity to narrow their focus. Key areas include visualization, big data, and neural computing. With a master-level degree in data science, graduates apply their expertise to tackle real-world data dilemmas. With cutting-edge know-how, professionals are trained to source data sets globally, extrapolating superior insights. Students acquire in-depth knowledge of machine learning, game theory, cryptology, and other technical subject matter to corporeality practicums.
Get more detailed information about the degree, and review data science specifics.
Master’s in Data Science vs Masters in Computer Science: Key Differences
When examining the core differences between a data science versus computer science master’s, it is helpful to study the general focal areas side by side, per the table below:
Computer Science | Data Science |
Software Engineering | Mathematical Data Science |
Cybersecurity | Artificial Intelligence |
Computational Theory | Probability Theory |
Programming Languages | Python Programming |
Database Systems | Big Data |
Algorithms | Stats & Statistical Analysis Methods |
Operating Systems | Reinforcement Learning |
The vision for both science fields is also unique and may embolden students to confidently choose between one or the other.
Some are guided by the words of Marc Andreessen, computer scientist and inventor of Netscape, “The spread of computers and the Internet will put jobs in two categories. People who tell computers what to do, and people who are told by computers what to do.” These technologists are motivated to operate entirely through the computer.
Others find inspiration in the words of Peter Sondergaard, data scientist and Senior Vice President of Garner Research, “Information is the oil of the 21st century, and analytics is the combustion engine.” Finding key, decision-influencing gems within millions of rows of customer data can be incredibly gratifying.
The decision between a master’s degree in data science versus computer science may be determined based on which quote and idea is most appealing to you.
Prerequisites
Before graduate program advancement, you should have completed a bachelors’ degree. There are college-level requirements of calculus, advanced math, and Java/Python coursework for both degrees. For computer science, additional data structures and computer organization experience are needed.
While the prerequisites are the same, the skill “must-haves” do vary. A computer science program appeals to students who have an affinity to the science disciplines, specifically subjects that call for applied analytics and logic.
In contrast, data science incorporates algorithms, database concepts, programming languages, and statistics. Whether contemplating a master’s in data science or computer science, an aptitude for math is imperative.
Curriculum
In both curriculums, students are often required to complete a total of 10 courses. Depending on the school, the general syllabus may be organized as follows:
# Required | # Elective | |
Computer Science | 3 |
|
Data Science | 7 |
|
The computer science curriculum is designed to a) foster a broad perspective of the general field and then b) take a deep dive into a concentration track. Areas of concentration may include but are not limited to independent research, theory, applications, systems, software, and reasoning.
The data science prospectus is typically comprised of foundation, required, and elective courses. Additionally, class titles range from cloud computing and network security to modern cryptography and computational genomics.
Skills
It is useful, when analyzing a computer science versus data science master’s, to hone in on the skill requirements. When deciding, consider the personal question, should I delve into what comes naturally or build on skills that I may not currently excel in?
Again, there is overlap, so review the respective attributes for each:
Computer Science | Data Science | |
Technical Mastery/Toolbox |
|
|
Professional Traits |
|
|
Specializations
Many students begin their master’s program knowing precisely their intended area of focus. Others need time to identify their targeted track. Specializations can be categorized as follows:
Computer Science
- Cyber Ops
- Cybersecurity
- Software Engineering
- Bioinformatics
- Artificial Intelligence
Data Science
- Stats
- Machine Learning
- Modeling
- Data Mining
- Business Intelligence
Faculty, researchers, and advisors are always available to help guide the process. Choosing the right specialization is important for future alignment with job prospects.
Career Options
Perhaps the most influential aspect in assessing the differences between the two fields of study is understanding the career titles of each path. Below is a sampling of the plethora of professional opportunities along with average median salaries taken from Glassdoor:
Computer Science
- Software Development Director ($179,417)
- Principal Software Engineer ($156,483)
- Site Reliability Engineer ($127,718)
- Network Security Consultant ($100,234)
- Development Operations Engineer ($131,680)
- Cybersecurity Engineer ($107,042)
- Security Engineer ($111,691)
- Full Stack Software Developer ($86,731)
Data Science
- Data Analyst ($69,517)
- Data Scientist ($117,212)
- Machine Learning Engineer ($131,001)
- Machine Learning Scientist ($137,053)
- Applications Architect ($129,000)
- Data Architect ($118,868)
- Infrastructure Architect ($127,676)
- Statistician ($88,989)
Whether students’ long-term plans are data-centric or computer-focused, undoubtedly, an exciting, stable career with high-earning potential is just around the corner.
Outlook
The outlook for working professionals in the computer and data science arena is exceedingly bright. The anticipated 22% job growth from 2020 to 2030 is much higher than average. The elevated levels of career opportunities can be attributed to the following key economic factors: demand, growth, and supply. Over-indexing in all three categories, well-educated professionals will have their choice in job functions and features.
From small business owners to enterprise tech giants, the adoption rate of digital corporate culture is off the charts. The percentage of job opportunities in the data science sphere has been cited as high as 417%. Notable as well is the substantial number of related jobs that require a master’s degree.
Science professionals are challenged with obtaining world-class education to remain current in the latest global techniques.
How to Decide Between a Master’s in Computer Science Versus a Master’s in Data Science
Choosing between a data science or computer science master’s appears similar from the periphery, but there are fundamental differences.
Both programs require an aptitude for math and critical thinking, but they vary in subject matter specifics. Computer science leans towards software engineering, cybersecurity, and networking. Comparatively, data science is focused on data mining, modeling, and machine learning.
There is enormous opportunity and demand for experienced computer professionals, engineers, and scientists who possess elevated levels of technical expertise. Job functions are also unique.
Choose what is most exhilarating to you personally and best aligns with your career goals. The knowledge acquired from a master’s degree in computer science or data science is applicable to almost every industry.
Learn more about Johns Hopkins Engineering for Professionals Computer Science Master’s program or our Data Science Master’s program.
Still, have questions? That is often the case when making a big decision. We welcome any questions to admissions.